The diagnostic process is important in medicine including the accuracy of tests and investigations in determining disease.
There are four circumstances in relation to a test / investigation and a disease / condition:
- Test is positive and the person has the condition
- Test is negative but the person has the condition
- Test is positive but the person does not have the condition
- Test is negative and the person does not have the condition.
This classification process often results from diagnostic tests and investigations in the medical field determining if a person has specific disease or medical condition or not. However, it can also be used in other circumstances, for example, from modelling from the ‘paired analysis’ which examined and modelled excess weight among children aged 10-11 years (in school Year 6) based on gender, deprivation and weight classification of the child six years earlier when they were aged 4-5 years (in reception year at school). The results from the statistical model can then be compared with if the child actually did have excess weight in Year 6 or not.
Tests will never be 100% accurate, and some tests will be better than others so it is important to compare some different measures around testing to determine if the test is ‘good’ or not.
The number in each of the four categories can be added to a table as follows:
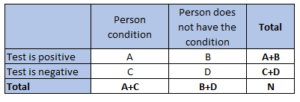
There are a number of measures that can be calculated from the table. A very good test would have high values for all of these measures, but in practice it is generally the case where there is a compromise on the different values for different tests and circumstances.
An example of such a test or statistical model and comparison with the ‘true’ situation can be found on the Children and Young People Healthy Weight, which examines the ‘accuracy’ of a statistical model of ‘predicting’ excess weight in school children in Year 6 (children aged 10-11 years) who were measured in 2022/23 based on their gender, deprivation score (based on where they live in Hull) and their weight classification in reception year (at age 4-5 years) six years earlier in 2016/17.
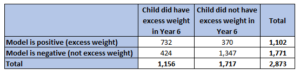
SENSITIVITY
This is the percentage of people with the condition who are correctly identified as such from the test (i.e. the test is positive).
It is calculated as 100 x A / (A+C).
“If a person has the condition, what is the likelihood the test will be positive?”
A test that is 100% sensitive means that all those with the condition will be picked up by the test. There will be no false negatives.
A sensitive test is used for excluding a condition as it rarely misclassifies a person with the disease as not having the condition / being healthy.
If sensitivity is low, it means that a high proportion of the people with the condition will have a negative test result. That is, the test will not pick them up, and they will be missed. So they will not get the treatment or intervention they need. If the disease has a high mortality rate, then it would be more important that the test has high sensitivity.
Sensitivity in the above table 63.3%. This means that out of those with excess weight in Year 6, the model would correctly identify 63.3% of them. However, this does mean that the model would not pick up 36.7% of the children with excess weight in Year 6. So if children at a higher risk (based on the model) of excess weight in Year 6 (‘positive’ for the model) were offered an intervention there would be a third of the children who needed the intervention who did not receive it.
SPECIFICITY
This is the percentage of people without the condition who are correctly identified as such from the test (i.e. the test is negative).
It is calculated as 100 x D / (B+D)
“If a person does not have the condition, what is the likelihood the test will be negative?”
A test that has 100% specificity means that all those without the condition will be correctly identified by the test. There will be no false positives.
A specific test is used for ruling out a condition as it rarely misclassifies a person without the disease as having the condition / being sick or ill.
If specificity is low, it means that a high proportion of the people without the condition will have a positive test result. That is, the test will suggest they have the condition when they do not. So if there are further tests, treatments or interventions for those who have the condition, you would be subjecting those who do not have the condition to unnecessary tests, treatments or interventions. This has ethical implications if the disease is serious or if further tests are invasive, as you will subjecting people to unnecessary worry and concern. Furthermore, there could be a high resource usage with implementing unnecessary further tests, treatments or interventions.
Specificity in the above table 78.5%. This means that out of those without excess weight in Year 6, the model would correctly identify 78.5% of them. However, this does mean that there would be a false positive rate of 21.6%. So if children at a higher risk (based on the model) of excess weight in Year 6 (‘positive’ for the model) were offered an intervention, then there would be a sizeable proportion of children receiving an intervention who didn’t need it which has implications on ethics and resources.
POSITIVE PREDICTIVE VALUE
This is the percentage of all those with a positive test result who have the condition.
It is calculated as 100 x A / (A+B)
“If the test is positive, what is the likelihood the person will have the condition?”
The positive predictive value is affected by the prevalence of the condition being examined (as well as on the sensitivity and specificity of the test). So if different tests are being compared, you must ensure that the same population is used or another population is used but it has the same underlying prevalence of the condition.
The lower the prevalence of true positives, the lower the proportion of true positives among those testing positive, therefore, the positive predictive value will be lower.
The positive predictive value in the above table is 66.4%. The test is correctly identifying around two-third of children who have excess weight in Year 6.
NEGATIVE PREDICTIVE VALUE
This is the percentage of all those with a negative test result who do not have the condition.
It is calculated as 100 x D / (C+D)
“If the test is negative, what is the likelihood the person will not have the condition?”
The negative predictive value is affected by the prevalence of the condition being examined (as well as on the sensitivity and specificity of the test). So if different tests are being compared, you must ensure that the same population is used or another population is used but it has the same underlying prevalence of the condition.
The negative predictive value in the above table is 76.1%. The test is correctly identifying around three-quarters of children who do not have excess weight in Year 6.
CHOICE BETWEEN TESTS
In many cases, the test may result in a value that is a range, e.g. a measure of something in the blood, and in these cases, it is possible to compare different cut-off values for the test to obtain the ‘best’ circumstances for the condition in relation to these statistical measures. The compromise will depend on the disease and the prevalence of the disease.
If the disease has a very high mortality rate and the test is cheap and non-invasive, and further more accurate expensive tests are available, then it may be that the you want sensitivity to be high and you compromise on other measures. This would mean that you miss very few people with the disease, i.e. the cheap tests picks them up. However, it probably will pick up a higher percentage of people without the disease, and you end up performing the more expensive accurate test on more people, but at least you are unlikely to miss anyone with the first test which you don’t want to do if the mortality rate is high. However, there will be other factors, such as the invasiveness of the second test and the very important consideration in relation to ethnics (subjecting people who probably don’t have the condition to extra tests and worry) as well as an impact on resource use (a high rate of expensive testing on people who probably don’t have the disease).
If the condition is not immediately serious and the intervention is not harmful and is cheap, then it might be that you don’t mind if specificity is low. For example, if you are predicting excess weight in Year 6 then you might not mind if the test or model has more positive results and picks up more cases of excess weight, but inadvertently at the same time results in more positive results where the person won’t have excess weight in Year 6 where the intervention is cheap, non-invasive and will not cause high levels of worry, such as with an intervention of sending out a letter to parents of children in Year R who are at a higher risk which contains useful information on reducing excess weight.
Reference: Cochrane UK. Sensitivity and specificity explained. https://uk.cochrane.org/news/sensitivity-and-specificity-explained-cochrane-uk-trainees-blog